

이 실습은 위의 자료를 참고하여 작성하였습니다. 실습은 colab에서 진행하였습니다.
Feast Tutorial
Common Issues
- Training-serving skew & complex data joins
- Online feature availability
- Feature resuability & model versioning
⇒ Feast를 이용하여 해결할 수 있다!!
Step 1 : Install Feast
pip 를 이용해서 Feast를 설치한다.
%%sh pip install feast -U -q pip install Pygments -q echo "Please restart your runtime now (Runtime -> Restart runtime). This ensures that the correct dependencies are loaded."
설치하고 나서 runtime을 꼭 restart 해주어야 한다.
Step 2 : Create a feature repository
feature repository 는 feature store에 담을 feature들을 생성하는 코드가 모여있는 곳이다.
먼저 feature_repo
라는 이름으로 feature repository를 생성한다.
!feast init feature_repo
그럼 위와 같이 feature_repo가 생성되며 안에 기본 샘플 파일들이 생성된 것을 볼 수가 있다.
feature repository의 구성 :
data/driver_stats.parquet
: parquet으로 저장되어 있는 raw data
driver_stats.parquet
index | event_timestamp | driver_id | conv_rate | acc_rate | avg_daily_trips | created |
---|---|---|---|---|---|---|
0 | 1005 | 0.9725654721260071 | 0.3631921708583832 | 410 | ||
1 | 1005 | 0.04274604469537735 | 0.2000729739665985 | 856 | ||
2 | 1005 | 0.9639862179756165 | 0.7615727186203003 | 691 | ||
3 | 1005 | 0.9086549878120422 | 0.7580361366271973 | 142 | ||
4 | 1005 | 0.433357298374176 | 0.9592541456222534 | 828 | ||
5 | 1005 | 0.8010686635971069 | 0.4016995131969452 | 697 | ||
6 | 1005 | 0.4435613453388214 | 0.8471459150314331 | 987 | ||
7 | 1005 | 0.4307439923286438 | 0.5900391340255737 | 520 | ||
8 | 1005 | 0.4524332880973816 | 0.6440556645393372 | 716 | ||
9 | 1005 | 0.7183521389961243 | 0.16217832267284393 | 985 | ||
10 | 1005 | 0.48318737745285034 | 0.215327188372612 | 831 |
feature_store.yaml
: featire repo가 어떻게 실행될 지 등의 설정 값이 작성된 파일
project: feature_repo registry: data/registry.db provider: local online_store: path: data/online_store.db
example.py
feature가 정의 되어있는 Python file
# This is an example feature definition file from google.protobuf.duration_pb2 import Duration from feast import Entity, Feature, FeatureView, FileSource, ValueType # Read data from parquet files. Parquet is convenient for local development mode. For # production, you can use your favorite DWH, such as BigQuery. See Feast documentation # for more info. driver_hourly_stats = FileSource( path="/content/feature_repo/data/driver_stats.parquet", event_timestamp_column="event_timestamp", created_timestamp_column="created", ) # Define an entity for the driver. You can think of entity as a primary key used to # fetch features. driver = Entity(name="driver_id", value_type=ValueType.INT64, description="driver id",) # Our parquet files contain sample data that includes a driver_id column, timestamps and # three feature column. Here we define a Feature View that will allow us to serve this # data to our model online. driver_hourly_stats_view = FeatureView( name="driver_hourly_stats", entities=["driver_id"], ttl=Duration(seconds=86400 * 1), features=[ Feature(name="conv_rate", dtype=ValueType.FLOAT), Feature(name="acc_rate", dtype=ValueType.FLOAT), Feature(name="avg_daily_trips", dtype=ValueType.INT64), ], online=True, batch_source=driver_hourly_stats, tags={}, )
위의 예제는 3개의 feature를 정의하였다. ( conv_rate
acc_rate
avg_daily_trips
)
Step 3: Deploy your feature store
example.py
에서 정의한 feature store를 배포하는 코드는 아래와 같다
!feast apply
이렇게 실행하고 나면 feture_store.yaml
에 설정된 값에 따라 다음처럼 추가적인 파일이 생긴다.
data/
내에 online_store.db
, registry.db
파일이 생긴 것을 알 수 있다. (이는 우리가 provider: local
로 설정해서 그렇다.)
Step 4: Generate training data
from datetime import datetime, timedelta import pandas as pd # The entity dataframe is the dataframe we want to enrich with feature values entity_df = pd.DataFrame.from_dict( { "driver_id": [1001, 1002, 1003], "label_driver_reported_satisfaction": [1, 5, 3], "event_timestamp": [ datetime.now() - timedelta(minutes=11), datetime.now() - timedelta(minutes=36), datetime.now() - timedelta(minutes=73), ], } )
feature store에서 feature를 불러와 위에 정의한 데이터 프레임에 추가를 한다.
from feast import FeatureStore store = FeatureStore(repo_path=".") training_df = store.get_historical_features( entity_df=entity_df, # 위에서 만든 데이터프레임을 넘겨준다. feature_refs = [ 'driver_hourly_stats:conv_rate', 'driver_hourly_stats:acc_rate', 'driver_hourly_stats:avg_daily_trips' ], # 불러올 feature를 적는다. ).to_df() training_df.head()
위에서 정의한 3개의 feature, conv_rate
, acc_rate
, avg_daily_trips
가 join된 것을 확인할 수 있다.
Step 5: Load features into your online store
이번에는 feature store에서 가장 최근 등록된 값들만 불러오자.
Using feast materialize-incremental
materialize-incremental
serializes all new features since the last materialize
call
from datetime import datetime !feast materialize-incremental {datetime.now().isoformat()}
materialize
를 실행하면 데이터가 online_store.db
와 registry.db
에 저장된다(?)
print("--- Data directory ---") !ls data import sqlite3 import pandas as pd con = sqlite3.connect("data/online_store.db") print("\n--- Schema of online store ---") print( pd.read_sql_query( "SELECT * FROM feature_repo_driver_hourly_stats", con).columns.tolist()) con.close()
Step 6: Fetching feature vectors for inference
from pprint import pprint from feast import FeatureStore store = FeatureStore(repo_path=".") feature_vector = store.get_online_features( features=[ "driver_hourly_stats:conv_rate", "driver_hourly_stats:acc_rate", "driver_hourly_stats:avg_daily_trips", ], entity_rows=[ {"driver_id": 1004}, {"driver_id": 1005}, ], ).to_dict() pprint(feature_vector)
feature store에 최근에 등록된 feature를 잘 가져온 것을 볼 수 있다.
정리
- 이 레포 안에서
feast
SDK와 파이썬을 이용하여 feature를 정의할 수 있다. 구체적으론 코드에서 다음을 정의한다.feast init <repo_name>
으로 사용할 feature store repository를 만들 수 있다.
DataSource
- 데이터를 가져올 데이터 소스를 지정한다.
- File, BigQuery 등이 되겠다.
Entity
- Feature 그룹의 대표 ID를 지정한다.
FeatureView
- 위에서 지정한
DataSource
,Entity
를 가지고 Feature Store에 저장할 Feature 그룹을 지정한다.
- 위에서 지정한
- 코드를 작성한 후
feast apply
로 feature store를 생성 및 업데이트할 수 있다.
feast materialize
로 최신 feature 값들을 저장 및 버전 관리할 수 있다.
- 머신러닝 코드에서
feast
SDK로 위 feature store에 정의한 feature 들을 가져올 수 있다.
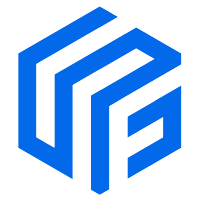
위의 블로그가 정리가 잘되어 있어서 참고를 많이 했습니다!
Uploaded by Notion2Tistory v1.1.0